Clarkson’s Farm and the Clarkson Ring
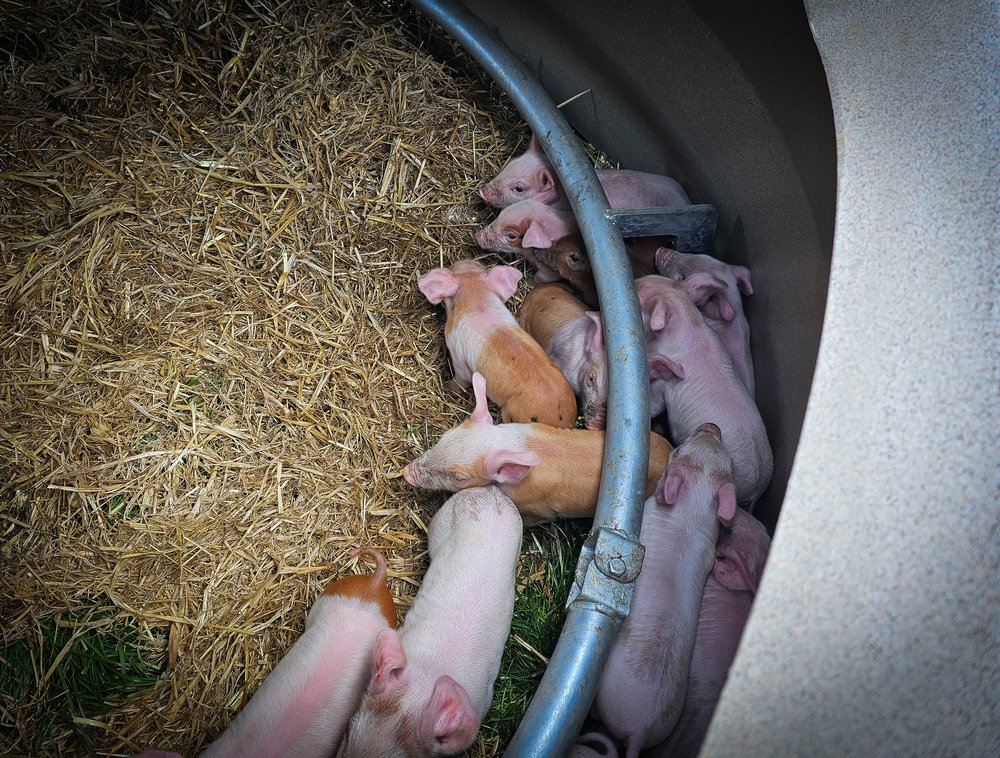
The hit series “Clarkson’s Farm” has Jeremy Clarkson attempt farming, in a highly amusing mix between Mr. Bean, John Cleese in Clockwise, and Pat & Mat. After binge-watching the first three seasons, I was struck by one particular topic: the mass death of the piglets. Clarkson houses his sows in “pigloos” — dome-shaped pig farrowing pens. The first time the…
read more