Bayes Factors for Stan Models without Tears
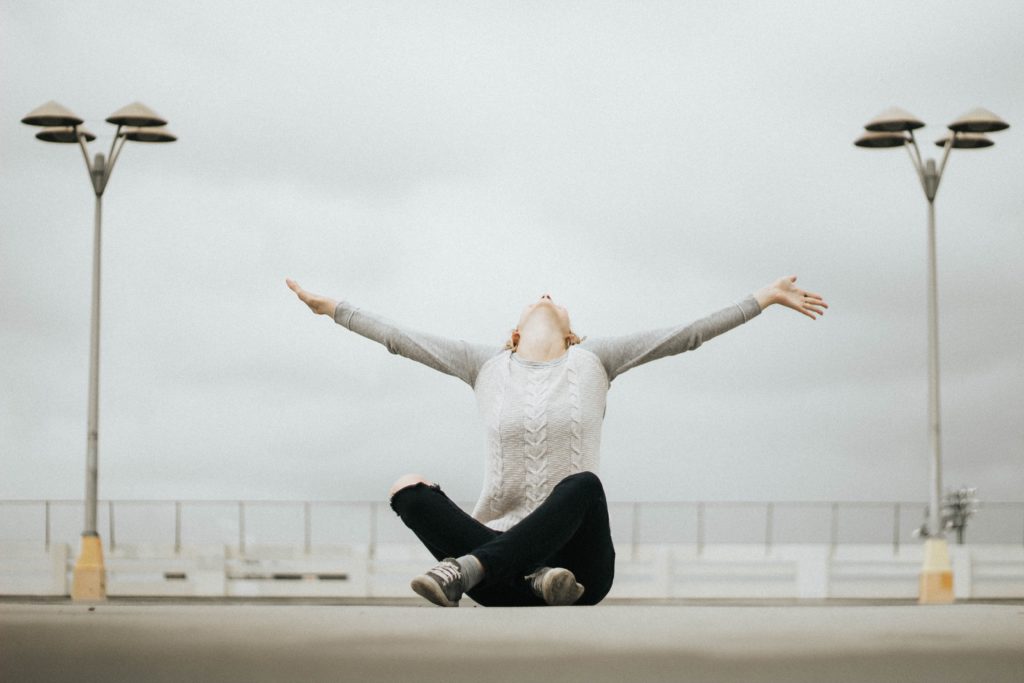
For Christian Robert’s blog post about the bridgesampling package, click here. Bayesian inference is conceptually straightforward: we start with prior uncertainty and then use Bayes’ rule to learn from data and update our beliefs. The result of this learning process is known as posterior uncertainty. Quantities of interest can be parameters (e.g., effect size) within a single statistical model or…
read more