Too Good to be True: A “Fake” Podcast on how to go from p-values to Bayes Factors
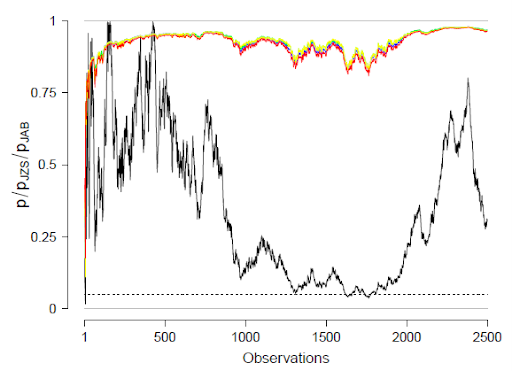
František Bartoš recently attended me to a podcast discussing my 3p√n paper (Wagenmakers, 2022). A podcast host and a statistician are discussing the contents of the paper for about 12 minutes; the link to the podcast is here. As I listened to the podcast, a few things stood out: The host and the statistician interpreted the contents of the paper…
read more