Bayesian Conference Next Week in Amsterdam: Advances in Network Modeling, Demography, and More
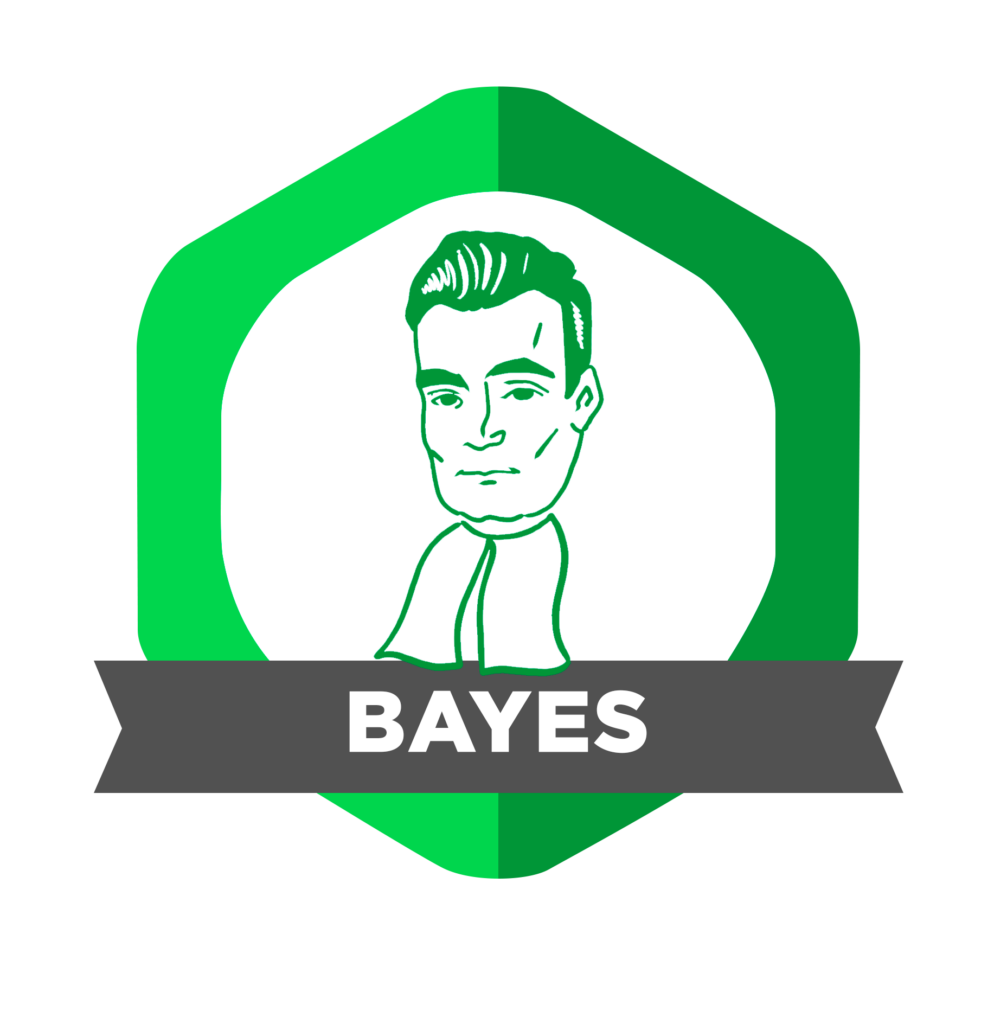
The conference “Bayesian Methods for the Social Sciences II” will take place October 16-18 (next week!) at the University of Amsterdam. Leading researchers will discuss Bayesian advances in network modeling, demography, model selection, and language change. The complete programme is here. You can register until just before the conference starts. Currently we still have enough room to accommodate a few…
read more