PROBABILITY DOES NOT EXIST (Part III): De Finetti’s 1974 Preface (Part I)
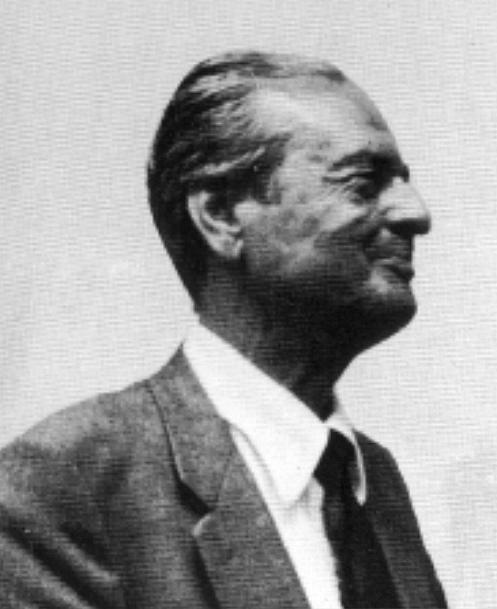
In an earlier blogpost I complained that the reprint of Bruno de Finetti’s masterpiece “Theory of Probability” concerns the 1970 version, and that the famous preface to the 1974 edition is missing. This blogpost provides an annotated version of this preface (de Finetti, 1974, pp. x-xiv). As the preface spans about four pages, it will take several posts to cover…
read more