The Galton board or quincunx is a fascinating device that provides a compelling demonstration of one the main laws of statistics. In the device, balls are dropped from above onto a series of pegs that are organized in rows of increasing width. Whenever a ball hits a particular peg, it can drop either to the right or to the left, presumably with a chance of 50% (but more about this later). When many balls are dropped, most of them remain somewhere near the middle of the device, but a few balls experience a successive run of movements in the same direction and therefore drift off to the sides.
Enough with the details. This year my wife bought me a Galton board with 3,000 balls and I’ve been messing around with it perhaps a little more than I should. By the way, I do not receive a penny from the good people at galtonboard.com, but I will say that at about $25 the board makes for a nice, geeky present. Here is one demonstration, in slow motion (a 32 MB file is here):
The Galton board is used most often to demonstrate the central limit theorem (or, more precisely, the De Moivre-Laplace theorem). The containers at the bottom indicate how many times a particular ball has moved to the left versus the right; this is a sum of many independent variables, and –approximately and asymptotically– this results in a Gaussian/normal distribution (for the Galton board, and for many other data-generating processes as well). My Galton board shown in the demo has the appropriate Gaussian/normal distribution painted across its containers.
After my wife saw me flip the Galton board back and forth a number of times she said “Why do you bother? The result is always the same”. Indeed, some people argue that the Galton board shows that even though the behavior of a single ball is unpredictable, the behavior of the ensemble is governed by iron statistical laws.
Model Misspecification
I wish to use the Galton board to make a different point. First, consider how the balls bounce around. According to the binomial model, each time a ball hits a peg, it should cleanly drop either to the left or to the right. But this is not what happens in our real-world Galton board. There, the balls bounce around wildly: they hit one another, they bounce upward, they hop to the side and hit the next peg in the same row, they ricochet off the walls, they skip several rows; a brief glance at the demonstration should convince anybody that the abstraction offered by the binomial model is not warranted — that is, the abstraction is clearly wrong and the model is misspecified. Nevertheless, the histograms at the bottom appear to be consistent with the binomial model — the normal distribution provides a good description of the end result. So there is considerable value to the use of a parametric model (e.g., the binomial model, or its normal approximation) even though we can be certain that the model is dead wrong in the details.
Incompleteness from Ignorance: Another Kind of Model Misspecification?
Now suppose we have a Galton board in which there does not appear to be any model-misspecification: balls are dropped one at a time, after a sufficiently long delay, so they do not bump into each other; the balls are made of soft material so that they do not jump up and hit other pegs, etc. But the binomial model is still not perfect. The binomial model assumes that each time a ball hits a peg, it goes left or right with probability 0.5. But this assumption is incorrect; the ball does not get to choose, somehow, whether it will go left or right — the ball is subject to the laws of physics; these laws determine whether the ball will go left or right, and the ball will obey these laws without fail. Despite first impressions, there is absolutely nothing random about the Galton board. Precise knowledge of the physical properties of the balls and the board would allow us, at least in principle, to predict the behavior of every single ball.
The same argument applies to statistical models generally. All statistical models involve noise, and this noise is an expression of our ignorance, not an expression of the true state of the world. There are some subtle arguments surrounding determinism and physics, in particular quantum mechanics (e.g., Becker, 2018; Earman, 1986) but in virtually all scenarios it is clear that if we know more about the data-generating process, we could substantially decrease the noise that is stipulated by our statistical model. In other words, our statistical models are by definition incomplete — they replace knowledge of the physical process by a catch-all component called “noise”, which reflects our ignorance about what is truly going on (see also our post on Jevons’ determinism). Of course, since we do not have infinite knowledge, a statistical model may be the best we can ever do. But the point is a principled one.
The binomial model for the idealized Galton board may be incomplete, but can we call it misspecified? I am leaning towards “yes”. Suppose both of us see the toss of a fair coin. I predict that the chance of it coming up tails is .50; you, however, have seen that the coin, just before it’s tossed, rests tails-up on the tosser’s thumb; this knowledge allows you to predict that the chance of it coming up tails is .51 (e.g., Diaconis, Holmes, & Montgomery, 2007). Is my model misspecified? Well, it misses important information and will predict worse than your model. This situation is similar to that of a regression model that omits a key predictor; such a model would generally be considered misspecified. It would, I think, be considered misspecified even if there was no way for the modeler of knowing the nature of the missing predictor — all that matters is that the model mismatches the data-generating process.
To elaborate, consider Jim, Johnny, and Jack who are standing side to side in front of a coin tossing machine. Their task is to predict the outcome of the next flip. Jim only knows the coin is fair, and he states the probability of the coin landing tails on the next flip is 50%. Johnny is taller than Jim and can just glimpse that the machine’s container has the coin “tails up”; Johnny uses this knowledge to make a more precise prediction, namely that the requested probability is 51%. Jack, however, is even taller than Johnny, so can also see the machine’s input panel that shows the set vertical force and angular momentum. This knowledge allows Jack to predict the outcome with much more certainty than Jim and Johnny. It seems to me that both Jim and Johnny’s models are misspecified; had they known what Jack knows, they would have incorporated that information and adjusted their predictions accordingly. But even Jack’s model is misspecified, in the sense that one may imagine a fourth person –James, say– who also knows the air resistance, which helps sharpen the prediction even more. This game of inventing hypothetical people who have access to additional informative predictors can continue until the chance of the next flip coming up tails is either 0 or 1. At that point, the model is no longer misspecified, but it has also stopped being statistical.
In sum, I am leaning towards the idea that statistical models are misspecified by definition: the noise that they stipulate is an implicit confession of ignorance, ignorance that can only be reduced by the introduction of more detailed knowledge of the data-generating process.
References
Becker, A. (2018). What is real? The unfinished quest for the meaning of quantum mechanics. London: John Murray.
Diaconis, P., Holmes, S., & Montgomery, R. (2007). Dynamical bias in the coin toss. SIAM Review, 49, 211-235.
Earman, J. (1986). A primer on determinism. Dordrecht: Reidel.
About The Author
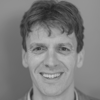