Karl Pearson’s Best Quotation?
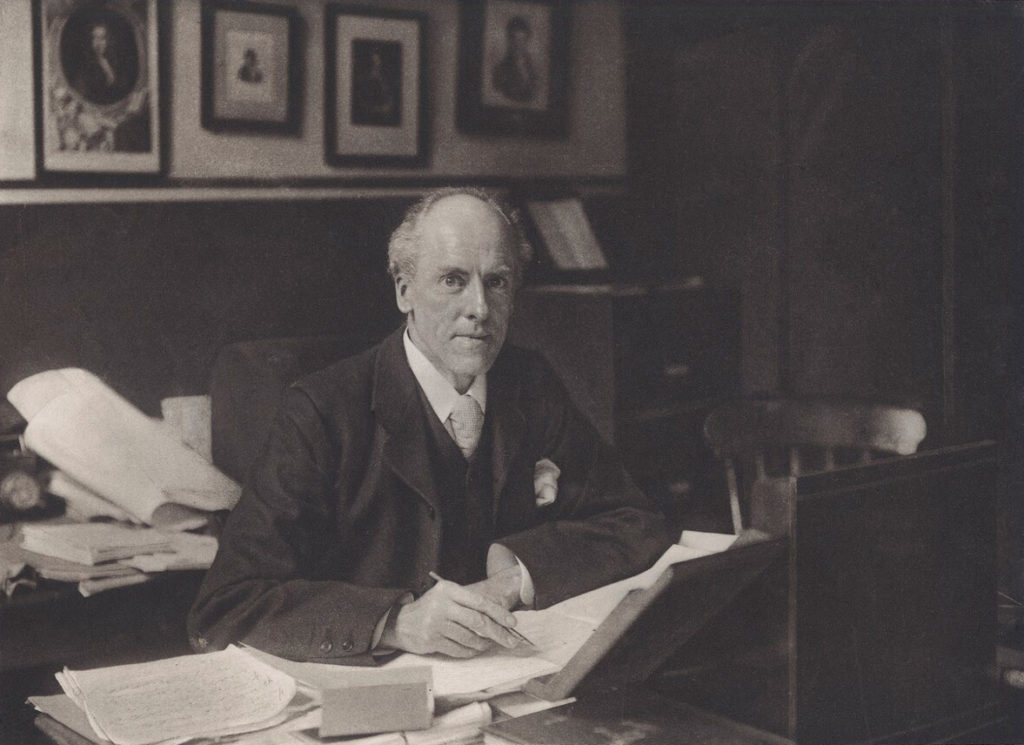
NB. The next post will discuss two of Karl Pearson’s worst quotations. “The field of science is unlimited; its material is endless, every group of natural phenomena, every phase of social life, every stage of past or present development is material for science. The unity of all science consists alone in its…
read more