Call for Crowdsourced Analysis: Many Analysts Religion Project (MARP)
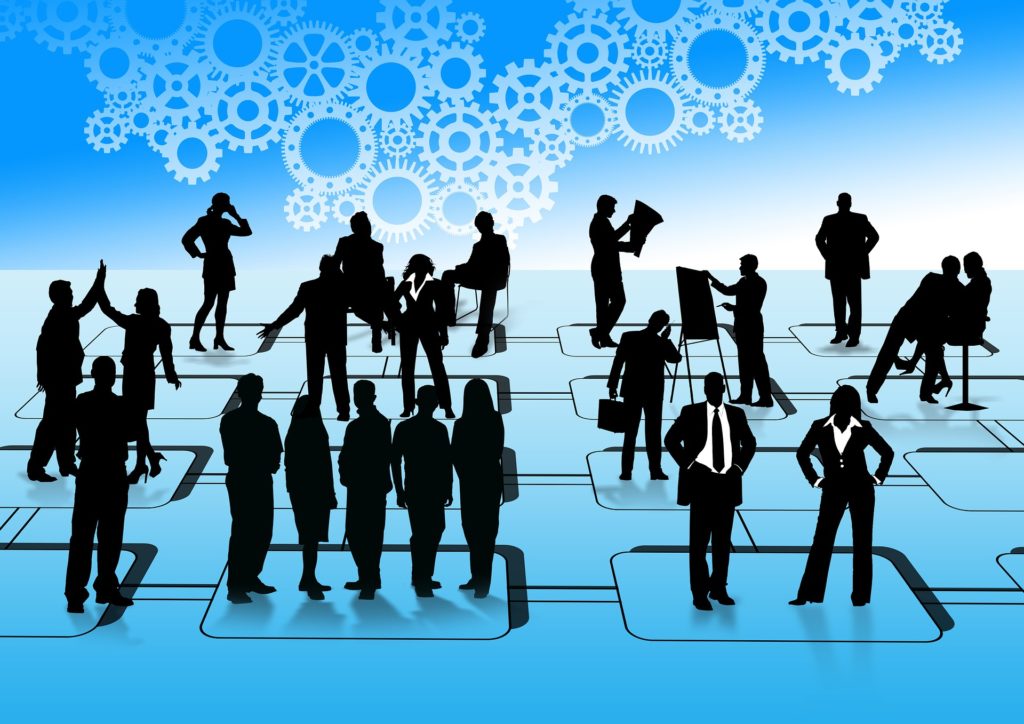
What is the idea? We invite researchers to answer two theoretically relevant research questions by analyzing a recently collected dataset on religion and well-being (N=10,535 from 24 countries). We aim to evaluate the relation between religiosity and well-being using a many analysts approach (cf. Silberzahn et al., 2018). This means that we are inviting multiple analysis teams to answer the…
read more