How to Evaluate a Subjective Prior Objectively
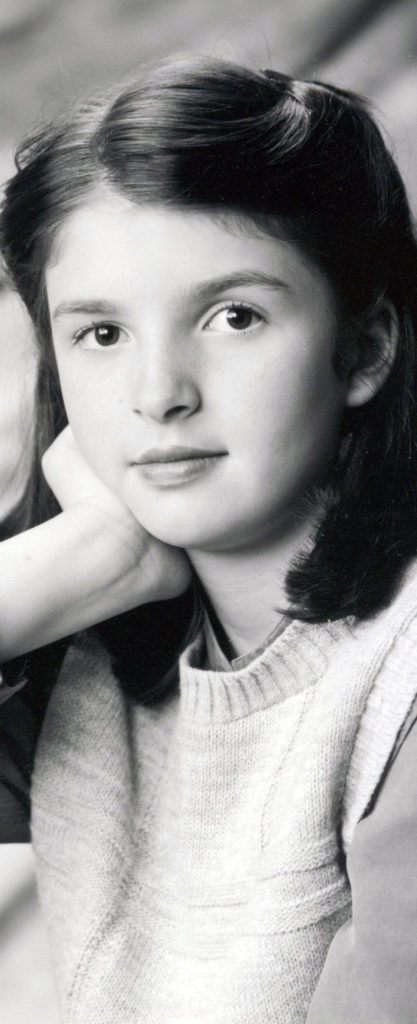
The Misconception Gelman and Hennig (2017, p. 989) argue that subjective priors cannot be evaluated by means of the data: “However, priors in the subjectivist Bayesian conception are not open to falsification (…), because by definition they must be fixed before observation. Adjusting the prior after having observed the data to be analysed violates coherence. The Bayesian system as derived…
read more