Karoline Huth is a first-year student in our Psychology Research Master at the University of Amsterdam. This blog post describes her presentation for the recent course on Bayesian Inference for Psychology. The assignment was to conduct a Bayesian reanalysis of existing data. Kudos to Karoline for being brave enough to share her work here! [EJ]
Presentation Intro and Background
Bayesian statistics is a trending topic in psychological research. Even though its benefits have been widely discussed (e.g., Marsman & Wagenmakers, 2017; Wagenmakers et al., 2018), many researchers still don’t use it. I argue these researchers fall in one of four subgroups:
- Those that are resistant: This group of researchers is the most difficult to address. They know exactly what the Bayesian approach is and how it works. But for whatever reason they stay resistant and prefer the common, frequentist approach.
- Those that are oblivious: There are those, unfortunately, that have never heard of the Bayesian approach. How is this possible? This group most likely consists of on the one hand inactive researchers and on the other students that are still solely taught the frequentist approach.
- Those that are lazy: Even though it has been their New-Year’s Resolution for the last five years, these researchers haven’t managed to learn more about the Bayesian approach and how to implement it. Consequently, they are not sufficiently confident to adopt the Bayesian framework.
- Those that are lost: Last of all, this group has heard of the Bayesian approach, is aware of its benefits, and knows the mathematical background. These researchers would like to apply the Bayesian approach but do not know what statistical software to use. In the end they resort to using the common frequentist analysis, saving them hours of programming.
This presentation tells the fictitious story of a lost researcher who was saved. We discuss an example from Moore et al. (2012) concerning patients with dementia who supposedly misbehave during the full moon. The story goes as follows: a researcher investigated whether the myth is true and the full moon creates a foul mood. This researcher would love to conduct a Bayesian analysis, but unfortunately lacks an appropriate tool. Luckily JASP came along with the mission to help fellows just like him. In the end the researcher was able to conduct his analyses and confidently state that the data are much more likely under the alternative hypothesis than the null hypothesis, supporting the assertion that patients with dementia show more disruptive behaviors during full moon.
Slides from the Presentation
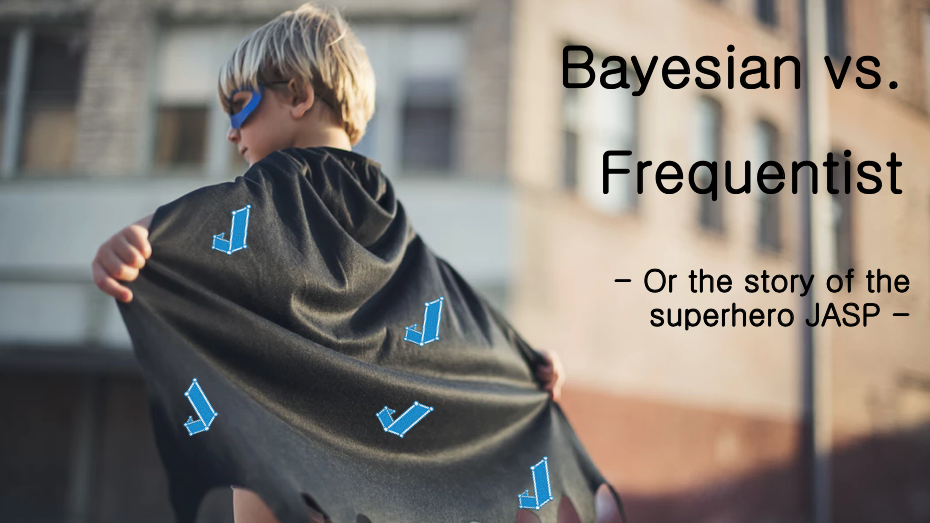
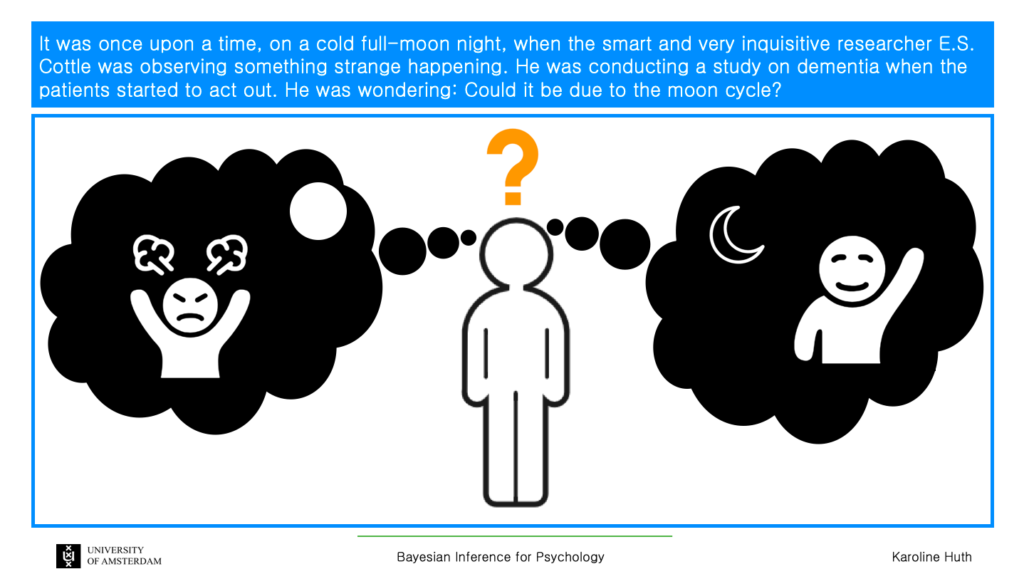
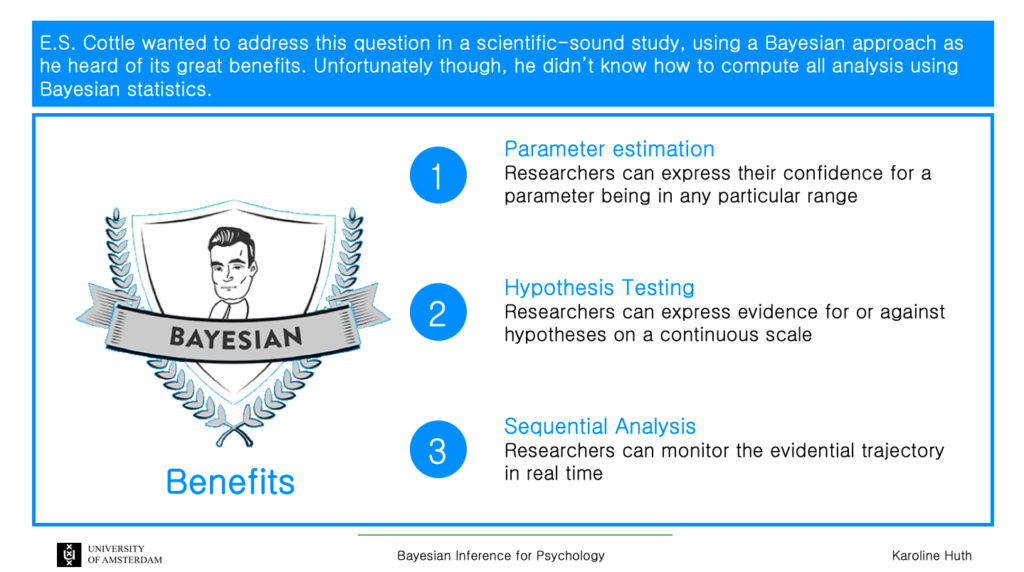
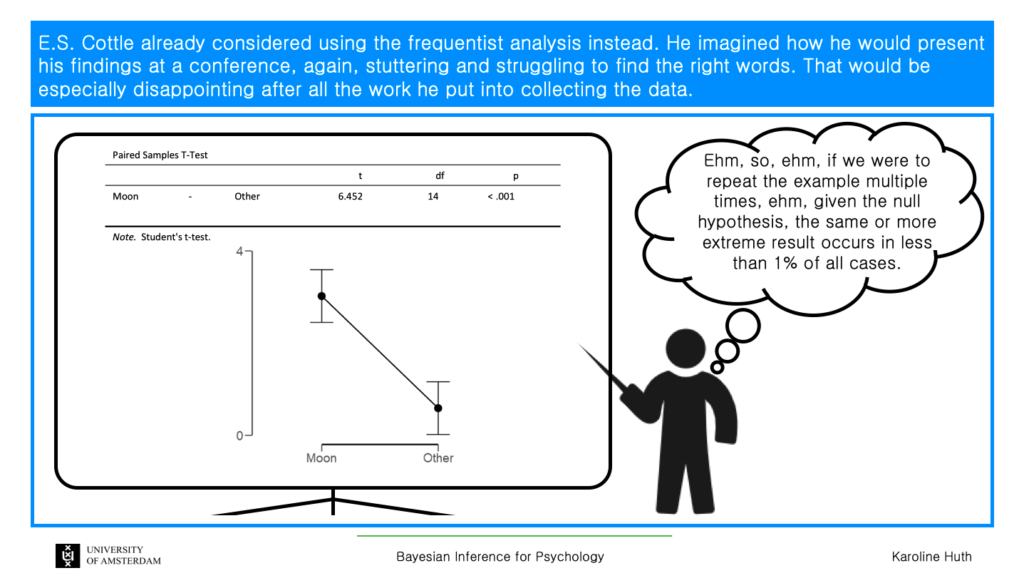
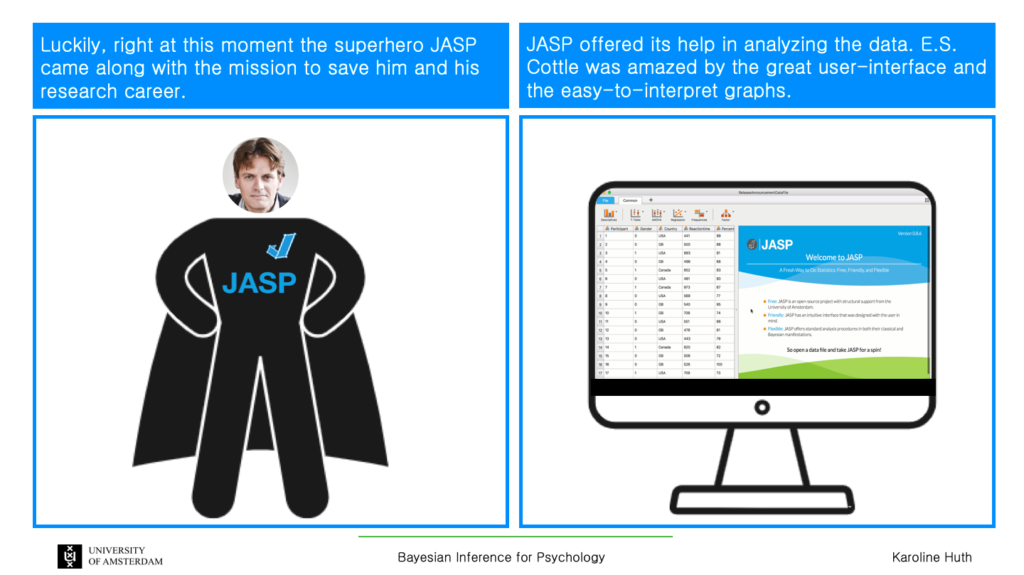
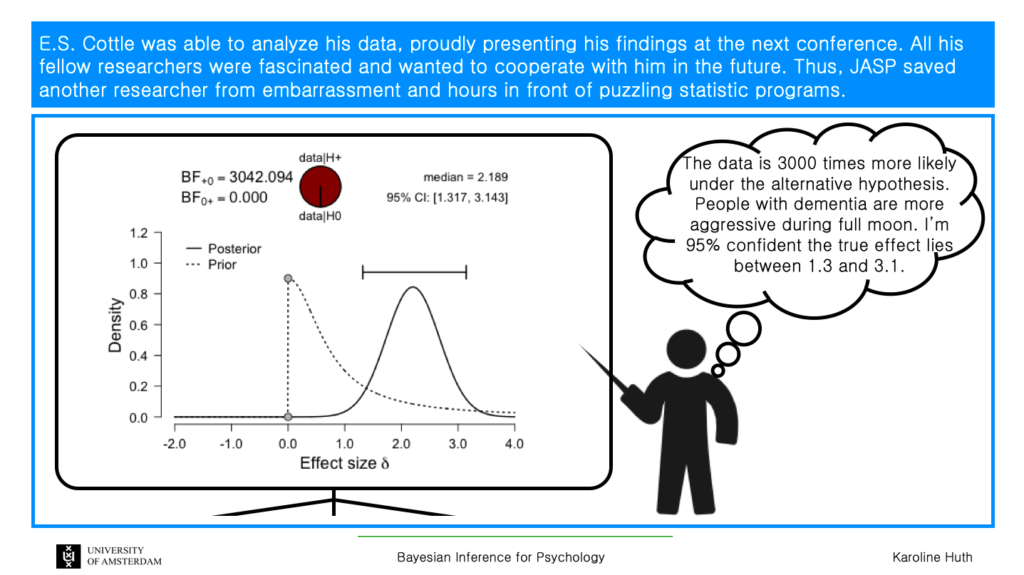
References
JASP Team (2018). JASP (Version 0.9)[Computer software].
Marsman, M., & Wagenmakers, E. J. (2017). Bayesian benefits with JASP. European Journal of Developmental Psychology, 14, 545-555.
Moore, D. S., McCabe, G. P., & Craig. B. A. (2012). Introduction to the Practice of Statistics (7th ed). New York: Freeman.
Wagenmakers, E. J., Marsman, M., Jamil, T., Ly, A., Verhagen, J., Love, J., … & Matzke, D. (2018). Bayesian inference for psychology. Part I: Theoretical advantages and practical ramifications. Psychonomic bulletin & review, 25, 35-57.
All icons are gratefully retrieved from the Noun Project (URL: https://thenounproject.com/).