Over the past year or so I’ve been working on a book provisionally titled “Bayesian bedtime stories”. Below is a part of the preface. This post continues the cooking analogy from the previous post.
Like cooking, reasoning under uncertainty is not always easy, particularly when the ingredients leave something to be desired. But unlike cooking, reasoning under uncertainty can be executed like the gods: flawlessly. The sacrifice that is required is only that one respects the laws of probability. Why would anybody want to do anything else?
This is not the place to bemuse the historical accidents that resulted in the rise, the fall, and the revival of Bayesian inference. But it is important to mention that Bayesian inference, godlike in its purity and elegance, is not the only game in town. In fact, researchers in empirical disciplines –psychology, biology, medicine, economics– predominantly use a different method to draw conclusions from data.
This alternative method is known as classical or frequentist, and those who adhere to it view probability as the rate with which something happens if it is attempted very often. For instance, the probability that a fair coin lands heads on the next toss is defined by considering the limiting proportion of times that the coin land heads if you were to throw it very many times.1 Moreover, frequentists believe the primary purpose of statistics is to use procedures that are reliable in the sense that they limit the proportion of erroneous conclusions in the long run. This has resulted in the development of concepts such as the -value,
-level, power, and confidence intervals. Some Bayesians believe that these frequentist concepts are nothing less than the work of Lucifer, Lord of Lies. Enticed by their simplicity and popularity among practitioners, many scientific projects have adopted frequentist methods only to reach conclusions that a more rational analysis would label premature and misleading. [see the earlier posts on Redefine Statistical Significance]
To clarify the peculiarity of the frequentist procedure, let us first revisit the cooking analogy. Confronted with six ounces of half-rotten meat, two old potatoes, and a molded piece of cheese, we have seen [in the previous post] that the Bayesian chef will produce a meal that cannot be improved upon, given the quality of the available ingredients. But what would a frequentist chef do? Well, the frequentist chef faces two serious problems.
The first problem is that for the frequentist chef, the cooking procedure itself is up for debate. As an example, consider the problem of estimating an interval for a binomial proportion; Brown et al. (2001) list eleven different methods, all with different properties. Among the eleven, the preferred method is…wait for it…there is no preferred method! Yes, there exist some general desiderata, some properties that a perfect estimator must have when considering its performance in repeated use. In repeated use, the perfect estimator needs to be consistent (so that it converges to the true value as sample size increases), it needs to be unbiased (so that it does not systematically over- or underestimate the true value), and it needs to have small variance (so that it yields precise estimates). But these desiderata are not laws, and in some situations biased estimators may be preferable to unbiased estimators. This means that the frequentist chef needs to make it up as he goes along, and there is no certainty that the procedure he happens to chose is superior over others.
The second problem that faces our frequentist chef is more fundamental: his cooking method is designed to achieve a particular performance on average, across repeated use. It is therefore insensitive to the details of the specific case. Assume that each day, the frequentist chef receives different ingredients (these represent the data); the chef will then apply a method of preparation that has ‘good coverage probability’ and that ‘controls the error rate’. For instance, the method of preparation may guarantee that no more than 5% of the produced meals produce indigestion. This method may consist of slightly overcooking the meat, mashing the potatoes, and throwing away half of the cheese. After all, it’s better to be safe than sorry. In repeated use, this method controls the error rate, but for specific cases, the method may nevertheless be ill-advised; one day the chef may by chance receive prime beef, high-quality potatoes, and a nice piece of Camembert — the safe method of preparation represents a wasted opportunity. Another day the chef may receive meat that is teeming with maggots — the safe method of preparation is still a recipe for disaster.
In sum, the Bayesian chef takes the ingredients and produces the best dish possible; the frequentist chef uses an ad-hoc method of preparation designed to work well on average, which means that for specific ingredients, the method can be shown to be wasteful or dangerous. Imagine two adjacent restaurants. The Bayesian restaurant has a sign that reads ‘We cook like the gods. Enjoy the perfect meal every day!’; the frequentist restaurant has a sign that reads ‘In the long run, no more than 5% of our meals give you indigestion!’ Where would sit down for dinner? A similar argument can be constructed for the judicial system — a judge’s sentence may either be godlike and refer to the individual case, or it can be based on performance in repeated use; clearly, judgments based on performance in repeated use can be silly and unjust for the specific case. The points above were underscored by Jaynes (1976, pp. 200-201):
“Our job is not to follow blindly a rule which would prove correct 90% of the time in the long run; there are an infinite number of radically different rules, all with this property. Our job is to draw the conclusions that are most likely to be right in the specific case at hand (…) To put it differently, the sampling distribution of an estimator is not a measure of its reliability in the individual case, because considerations about samples that have not been observed, are simply not relevant to the problem of how we should reason from the one that has been observed. A doctor trying to diagnose the cause of Mr. Smith’s stomachache would not be helped by statistics about the number of patients who complain instead of a sore arm or stiff neck. This does not mean that there are no connections at all between individual case and long-run performance; for if we have found the procedure which is `best’ in each individual case, it is hard to see how it could fail to be `best’ also in the long run (…) The point is that the converse does not hold; having found a rule whose long-run performance is proved to be as good as can be obtained, it does not follow that this rule is necessarily the best in any particular individual case. One can trade off increased reliability for one class of samples against decreased reliability or another, in a way that has no effect on long-run performance; but has a very large effect on performance in the individual case.”
Despite these and other complaints2, it is nevertheless true that in applied, run-of-the-mill statistical applications the frequentist school dominates. When students take a statistics course in biology, medicine, or the social sciences, it is almost certain that they will be taught frequentist methodology, and only frequentist methodology. They might not even be told that there exists another school. Sad!
Footnotes
1 The throws are hypothetical: the coin does not wear down over time.
2 A recent overview is provided in Wagenmakers et al. (2018) and Diaconis & Skyrms (2018).
References
Brown, L. D., Cai, T. T., & DasGupta, A. (2001). Interval estimation for a binomial proportion. Statistical Science, 16, 101-133.
Diaconis, P., & Skyrms, B. (2018). Ten Great Ideas About Chance. Princeton: Princeton University Press.
Jaynes, E. T. (1976). Confidence intervals vs Bayesian intervals. In Harper, W. L., & Hooker, C. A. (Eds.), Foundations of Probability Theory, Statistical Inference, and Statistical Theories of Science, Vol. II., pp. 175-257. Dordrecht, Holland: D. Reidel Publishing Company.
Wagenmakers, E.-J., Marsman, M., Jamil, T., Ly, A., Verhagen, A. J., Love, J., Selker, R., Gronau, Q. F., Smira, M., Epskamp, S., Matzke, D., Rouder, J. N., & Morey, R. D. (2018). Bayesian inference for psychology. Part I: Theoretical advantages and practical ramifications. Psychonomic Bulletin & Review, 25, 35-57. Open Access.
About The Author
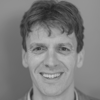