Background: the recent paper “Redefine Statistical Significance” suggested that it is prudent to treat p-values just below .05 with a grain of salt, as such p-values provide only weak evidence against the null. The counterarguments to this proposal were varied, but in most cases the central claim (that p-just-below-.05 findings are evidentially weak) was not disputed; instead, one group of researchers (the Abondoners) argued that p-values should simply be undervalued or replaced entirely, whereas another group (the Justifiers) argued that instead of employing a pre-defined threshold α for significance (such as .05, .01, or .005), researchers should justify the α used.
The argument from the Justifiers sounds appealing, but it has two immediate flaws (see also the recent paper by JP de Ruiter). First, it is somewhat unclear how exactly the researcher should go about the process of “justifying” an α (but see this blog post). The second flaw, however, is more fundamental. Interestingly, this flaw was already pointed out by William Rozeboom in 1960 (the reference is below). In his paper, Rozeboom discusses the trials and tribulations of “Igor Hopewell”, a fictional psychology grad student whose dissertation work concerns the study of the predictions from two theories, and
. Rozeboom then proceeds to demolish the position from the Justifiers, almost 60 years early:
“In somewhat similar vein, it also occurs to Hopewell that had he opted for a somewhat riskier confidence level, say a Type I error of 10% rather than 5%,
would have fallen outside the region of acceptance and
would have been rejected. Now surely the degree to which a datum corroborates or impugns a proposition should be independent of the datum-assessor’s personal temerity. [italics ours] Yet according to orthodox significance-test procedure, whether or not a given experimental outcome supports or disconfirms the hypothesis in question depends crucially upon the assessor’s tolerance for Type I risk.” (Rozeboom, 1960, pp. 419-420)
To drive the point home, imagine three brothers, Igor, Michael, and Boris, who study whether people can tell the difference between “Absolut vodka” and “Stolichnaya” (the null hypothesis is that people cannot tell the difference). Each of the brothers conducts an experiment with 100 participants. The brothers, however, have different levels of personal temerity. Igor uses α = .05, Michael uses α = .09, and Boris uses α = .001. By a remarkable coincidence, the three experiments yield exactly the same data, and p=.049. Clearly the data provide exactly the same level of support, the same evidence, and necessitate the same update in knowledge. In particular, the p-just-below-.05 result remains evidentially uncompelling, regardless of whether the data were collected by Igor, Michael, or Boris. “Justifying” a level of .05 does not turn weak evidence into strong evidence.
Remarkably, it has been claimed that scientists are not (or should not be) interested in learning from data, that is, in having data update knowledge. Instead, statisticians such as Neyman and Pearson proposed that scientists care mostly about making all-or-none “decisions”. We personally don’t believe this — of course scientists want to learn about the world when they conduct their experiments. The knowledge obtained may then be used to make decisions, if decisions need to be made, but the primary purpose is always learning. Rozeboom discusses this point in style:
“The null-hypothesis significance test treats ‘acceptance’ or ‘rejection’ of a hypothesis as though these were decisions one makes. But a hypothesis is not something, like a piece of pie offered for dessert, which can be accepted or rejected by a voluntary physical action. Acceptance or rejection of a hypothesis is a cognitive process, a degree of believing or disbelieving which, if rational, is not a matter of choice but determined solely by how likely it is, given the evidence, that the hypothesis is true.” (Rozeboom, 1960, pp. 422-423)
Rozeboom then continues and discusses the fallacy that making decisions (e.g., to conduct a follow-up experiment; to submit the result for publication, etc.) is ultimately what researchers are interested in:
“It might be argued that the NHD [the standard null-hypothesis decision procedure] test may nonetheless be regarded as a legitimate decision procedure if we translate ‘acceptance (rejection) of the hypothesis’ as meaning ‘acting as though the hypothesis were true (false).’ And to be sure, there are many occasions on which one must base a course of action on the credibility of a scientific hypothesis. (Should these data be published? Should I devote my research resources to and become identified professionally with this theory? Can we test this new Z bomb without exterminating all life on earth?) But such a move to salvage the traditional procedure only raises two further objections, (a) While the scientist—i.e., the person—must indeed make decisions, his science is a systematized body of (probable) knowledge, not an accumulation of decisions. The end product of a scientific investigation is a degree of confidence in some set of propositions, which then constitutes a basis for decisions.” (Rozeboom, 1960, p. 423)
The entire Rozeboom paper is well-worth reading, as his entire paper calls into question the idea of conducting experiments in order to make all-or-none decisions. As a pessimistic aside, we do not believe that Rozeboom-style arguments, however beautifully phrased, will convince people to abandon p-values or redefine their α-levels. The few remaining p-value apologists will never be convinced of the error of their ways, not even if Fisher, Neyman, and Pearson came back from the grave to coauthor a paper entitled “We Were Wrong and We’re Sorry: Bayesian Inference is the Only Correct Method for Inference”. Nor will abstract arguments convince the hordes of statistical practitioners — their primary goal is to convince reviewers, and any method whatsoever will be used once journal editors start demanding it (one case in point being the temporary adoption by Psychological Science of “p-rep”).
What does convince statistical practitioners, in our opinion, are concrete demonstrations of the benefits and feasibility of alternative procedures. Providing such demonstrations is of course one of the primary goals of this blog.
References
Rozeboom, W. W. (1960). The fallacy of the null-hypothesis significance test. Psychological Bulletin, 57, 416-428.
About The Authors
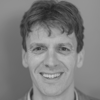
Eric-Jan Wagenmakers
Eric-Jan (EJ) Wagenmakers is professor at the Psychological Methods Group at the University of Amsterdam.
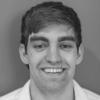
Quentin Gronau
Quentin is a PhD candidate at the Psychological Methods Group of the University of Amsterdam.