This post is a teaser for Sarafoglou, A., Haaf, J. M., Ly, A., Gronau, Q. F., Wagenmakers, E.-J., & Marsman, M. (2020). Evaluating multinomial order restrictions with bridge sampling. Preprint available on PsyArXiv: https://psyarxiv./bux7p/
Summary
Hypotheses concerning the distribution of multinomial proportions typically entail exact equality constraints that can be evaluated using standard tests. Whenever researchers formulate inequality constrained hypotheses, however, they must rely on sampling-based methods, such as the encompassing prior approach (Gu, Mulder, Deković, & Hoijtink, 2014; Klugkist, Kato, & Hoijtink, 2005; Hoijtink, Klugkist, & Boelen, 2008; Hoijtink, 2011) and the conditioning method (Mulder et al., 2009; Mulder, 2014, 2016). These methods, although popular and relatively straightforward in their implementation, are relatively inefficient and computationally expensive. To address this problem we developed a bridge sampling routine that allows an efficient evaluation of multinomial inequality constraints. An empirical application showcases that bridge sampling outperforms current Bayesian methods, especially when relatively little posterior mass falls in the restricted parameter space. The method is extended to mixtures between equality and inequality constrained hypotheses.
Example: Memory of Negative Life Events
Consider the study conducted by Uhlenhuth et al. (1974), who surveyed 735 adults to investigate the association between symptoms of mental disorders and experienced life stress. To measure participants’ life stress, the authors asked them to indicate, out of a list of negative life events, life stresses, and illnesses, which event they had experienced during the last 18 months prior to the interview. A subset of these data was reanalyzed by Haberman (1978, p. 3) who noted that retrospective surveys tend to fall prey to the fallibility of human memory, causing participants to report primarily those negative events that happened most recently. He, therefore, investigated the 147 participants who reported only one negative life event and tested whether the frequency of the reported events was equally distributed over the 18 month period. However, Haberman did not directly test the ordinal pattern implied by his assumption of forgetting, namely that the number of reported negative life events decreases as a function of the time passed. Figure 1 shows the frequency of reported negative life events in Haberman’s sample.
Figure 1. Frequency of reported negative life events over the course of the 18 months prior to the interview for Haberman’s (1978) sample of the data collected by Uhlenhuth et al. (1974). |
To test whether the reported negative life events decrease over time as a function of forgetting, we conduct a Bayesian reanalysis of Haberman’s sample. We test this inequality-constrained hypothesis Hr against the encompassing hypothesis He without constraints:
Hr : θ1> θ2 > … > θ18
He : θ1, θ2 , … , θ18 ,
where k denotes the probability of reporting a negative life event in month k.
Method
Using this empirical example, we investigate the precision and efficiency off the bridge sampling routine, the conditioning method, and the encompassing prior approach. We computed Bayes factors in favor of Hr 100 times for the same data set and for each estimation method and recorded the respective values and the runtime to produce a result. We assigned a uniform prior distribution to our parameters of interest, such that we could compute the prior probability of the constraint analytically.
Results
The estimated Bayes factors BFre are displayed in Figure 2. Bayes factors based on the bridge sampling method and the conditioning method are centered around the same value (M = 168.88 and M = 168.55, respectively); however, the bridge sampling estimates varied far less (SD = 1.873) than the estimates produced by the conditioning method (SD = 22.23).
The encompassing prior approach failed to estimate any Bayes factor, that is, for each iteration none of the 5 million posterior draws were in accordance with the constraint. This is not too surprising; the prior probability of samples obeying the constraint is already 1.3 billion times lower than the number of posterior samples drawn 1/118!. Thus, for the present example, the encompassing prior approach can be applied only with great investment of time.
Figure 2. Bayes factors for the bridge sampling method (black), the conditioning method (dark grey), and the encompassing prior approach (light grey) for the test of an order-restriction in Haberman’s (1978) data on the reporting of negative life events. Each dot represents one Bayes factor estimate in favor of Hr obtained by the respective method. The bridge sampling method yields more precise Bayes factor estimates than the conditioning method; the encompassing prior approach fails to estimate any Bayes factor. |
The computation times are displayed in Figure 3. Regarding the computational efficiency, the bridge sampling method had the lowest runtimes with a mean of M = 29.11 (SD = 0.39) seconds. The encompassing prior approach had comparable runtimes (M = 35.89, SD = 0.22). The conditioning method required the most time, with mean runtimes of M = 375.84 (SD = 5.04) seconds, which is more than 6 minutes to estimate one Bayes factor, compared to less than half a minute for the bridge sampling method.
Figure 3. Runtime for the bridge sampling method (black) is similar to that of the encompassing prior approach (light grey), whereas the conditioning method (dark grey) has much higher computational costs. However, even though the runtime for the bridge sampling method and the encompassing prior approach is similar, the latter method failed to estimate any Bayes factors. |
In sum, the empirical example demonstrates that the bridge sampling routine outperforms both the conditioning method and the encompassing prior approach. The bridge sampling estimates are considerably more precise than those of the conditioning method, and are obtained more quickly. The encompassing prior approach fails to estimate any Bayes factor altogether.
This example also illustrates how vulnerable the encompassing prior approach is to an increase in model size: even though the data strongly supported the inequality-constrained hypothesis over the encompassing hypothesis, none of 5 million posterior draws across 100 replications (for a total of 500 million draws) obeyed all of the inequality constraints. Note that, if for any replication a single posterior draw had obeyed the restriction (i.e., 1 out of 5 million) the estimated Bayes factor in favor of the inequality-constrained hypothesis would have been 1.28 x 109 (i.e., a staggering overestimate), as the prior probability of a sample obeying the restriction is minuscule.
References
Gu, X., Mulder, J., Dekovic, M., & Hoijtink, H. (2014). Bayesian evaluation of inequality constrained hypotheses. Psychological Methods, 19, 511-527.
Haberman, S. J. (1978). Analysis of qualitative data: Introductory topics (Vol. 1). Academic Press.
Hoijtink, H. (2011). Informative hypotheses: Theory and practice for behavioral and social scientists. Boca Raton, FL: Chapman & Hall/CRC.
Hoijtink, H., Klugkist, I., & Boelen, P. (Eds.). (2008). Bayesian evaluation of informative hypotheses. New York: Springer Verlag.
Klugkist, I., Kato, B., & Hoijtink, H. (2005). Bayesian model selection using encompassing priors. Statistica Neerlandica, 59, 57–69.
Mulder, J. (2014). Prior adjusted default Bayes factors for testing (in) equality constrained hypotheses. Computational Statistics & Data Analysis, 71, 448–463.
Mulder, J. (2016). Bayes factors for testing order–constrained hypotheses on correlations. Journal of Mathematical Psychology, 72, 104–115.
Mulder, J., Klugkist, I., van de Schoot, R., Meeus, W. H. J., Selfhout, M., & Hoijtink, H. (2009). Bayesian model selection of informative hypotheses for repeated measurements. Journal of Mathematical Psychology, 53, 530–546.
Sarafoglou, A., Haaf, J. M., Ly, A., Gronau, Q. F., Wagenmakers, E.-J., & Marsman, M. (2020). Evaluating multinomial order restrictions with bridge sampling. Preprint available on PsyArXiv: https://psyarxiv.com/bux7p/
Uhlenhuth, E. H., Lipman, R. S., Balter, M. B., & Stern, M. (1974). Symptom intensity and life stress in the city. Archives of General Psychiatry, 31, 759–764.
About The Authors
Alexandra Sarafoglou
Alexandra Sarafoglou is a PhD candidate at the Psychological Methods Group at the University of Amsterdam.
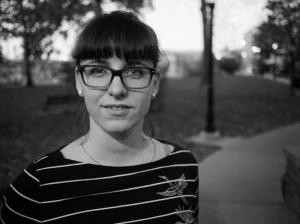
Julia Haaf
Julia Haaf is an assistant professor at the Psychological Methods Group at the University of Amsterdam.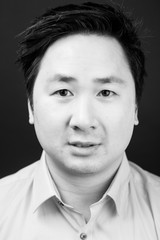
Alexander Ly
Alexander Ly is a postdoc at the Psychological Methods Group at the University of Amsterdam.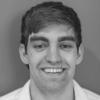
Quentin F. Gronau
Quentin is a PhD candidate at the Psychological Methods Group of the University of Amsterdam.
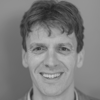
Eric-Jan Wagenmakers
Eric-Jan (EJ) Wagenmakers is professor at the Psychological Methods Group at the University of Amsterdam.
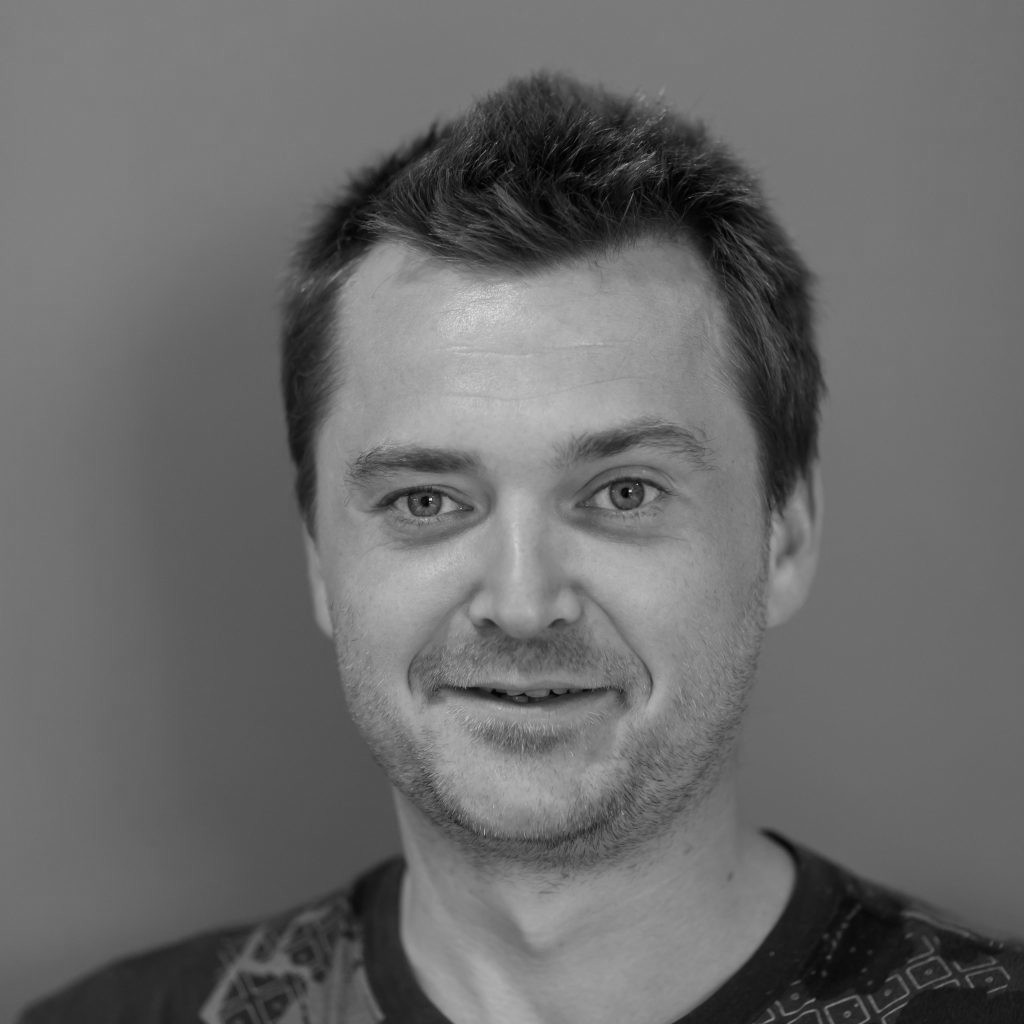